Os relatórios de PPC sempre foram essenciais e frustrantes. É essencial manter os clientes engajados informando-os sobre os resultados que você está gerando.
Mas também é frustrante por causa das discrepâncias de dados, análises complicadas e o tempo necessário para compartilhar relatórios compreensíveis e sem jargões com diferentes partes interessadas.
Felizmente, a IA está transformando esses obstáculos em oportunidades ao preencher lacunas deixadas pelo rastreamento em conformidade com a privacidade, revelando insights ocultos em conjuntos de dados impressionantes e automatizando relatórios para atender às necessidades de todas as partes interessadas.
Neste artigo, explicarei algumas das tecnologias usadas pelos profissionais de marketing modernos e compartilharei exemplos de como usei IA para otimizar meus relatórios de PPC.
1. Colete dados PPC completos e de alta qualidade
Precisamos de dados para nos orientar antes de otimizar contas e compartilhar nossas vitórias, então vamos começar por aí.
Os problemas com dados antes da IA
Dados inconsistentes e ausentes prejudicam os esforços de PPC.
Google, Meta, Microsoft e Amazon operam em seus próprios silos, cada um levando o crédito por todas as conversões que têm algum ponto de contato com suas plataformas. Isso leva à contagem dupla, dificultando decidir onde alocar orçamentos para resultados ideais.
Em outras palavras, os dados entre as várias plataformas de anúncios são inconsistentes. Especificamente, o valor de conversão que os anunciantes veem em seus dados comerciais pode ser menor do que a soma de todos os valores de conversão relatados pelas plataformas de anúncios.
Adicione a isso o desafio de dados ausentes. Regulamentações de privacidade como GDPR e as mudanças do iOS da Apple limitam as capacidades de rastreamento, o que causa perda de dados, caminhos de conversão incompletos e lacunas na atribuição.
Os profissionais de marketing que dependem muito do rastreamento baseado em pixels ou de cookies de terceiros, que se tornaram pouco confiáveis devido a restrições do navegador e cancelamentos de uso pelos usuários, observam um declínio contínuo na qualidade dos dados necessários para operar.
Embora a IA não possa nos fornecer dados perfeitos magicamente, ela pode preencher lacunas e restaurar insights, então vamos dar uma olhada em algumas das soluções nesse espaço.
Soluções baseadas em IA para higiene e conformidade de dados
1. Salas limpas de dados e medição de privacidade em primeiro lugar
Salas limpas como a Amazon Marketing Cloud (AMC) e o Google Ads Data Hub permitem que os anunciantes analisem com segurança dados anônimos de desempenho entre canais sem violar as leis de privacidade.
Essas plataformas agregam dados de diversas fontes, dando aos profissionais de marketing uma visão abrangente da jornada do cliente.
Exemplo:
Uma marca de varejo pode usar o AMC para avaliar como seus anúncios do Google e do Facebook influenciam as compras na Amazon. Com base no que eles descobrem, eles podem realocar orçamentos entre plataformas para maximizar o retorno geral sobre o investimento (ROI).
As salas limpas em si não são uma inovação de IA; no entanto, elas se beneficiam significativamente de vários recursos de IA.
Por exemplo, o Advantage+ da Meta usa insights de salas limpas para criar públicos semelhantes, mantendo a conformidade com a privacidade.
2. Conversões modeladas
Embora salas limpas sejam ótimas para unificar dados entre plataformas, sua utilidade depende da integridade dos dados.
When privacy regulations make it impossible to get all the data, clean rooms like Google Ads Data Hub and Amazon Marketing Cloud use AI-powered modeled conversions to estimate user journeys that can’t be fully tracked.
Modeled data is also used by tools like Smart Bidding, which leverages machine learning to predict conversions for users who opted out of tracking.
For users who opt out of tracking, Consent Mode still allows the collection of anonymized signals, which machine learning models can then use to predict conversion likelihood.
Example:
Google’s Smart Bidding leverages machine learning to optimize bids for conversions or conversion value.
In cases where conversion data is incomplete due to user consent choices or other factors, Smart Bidding can use modeled conversions to fill in gaps and make good bidding decisions.
The models do this by identifying patterns and correlations between user attributes, actions, and conversion outcomes.
While modeled conversions offer significant benefits in their ease of use (they’re basically provided without any extra effort by the ad platforms), it’s important to remember that they are only estimates and may not be perfectly accurate in all cases.
Advertisers should consider using modeled conversions in conjunction with other ways to get a more complete picture of campaign performance.
For example, advertisers can use Media Mix Models (MMM), a Marketing Efficiency Ratio (MER), or incrementality lift tests to validate that the data they are using is directionally correct.
3. Server-Side Tagging And First-Party Data Integration
Server-side tagging lets marketers control data collection on their servers, bypassing cookie restrictions.
Platforms like Google Tag Manager now support server-side implementations that improve tracking accuracy while maintaining privacy compliance.
Server-side tagging captures anonymous pings even when cookies are declined, feeding better signals into Google’s AI models for more accurate conversion modeling.
This gives AI more complete data when doing things like data-driven attribution (DDA) or automated bidding.
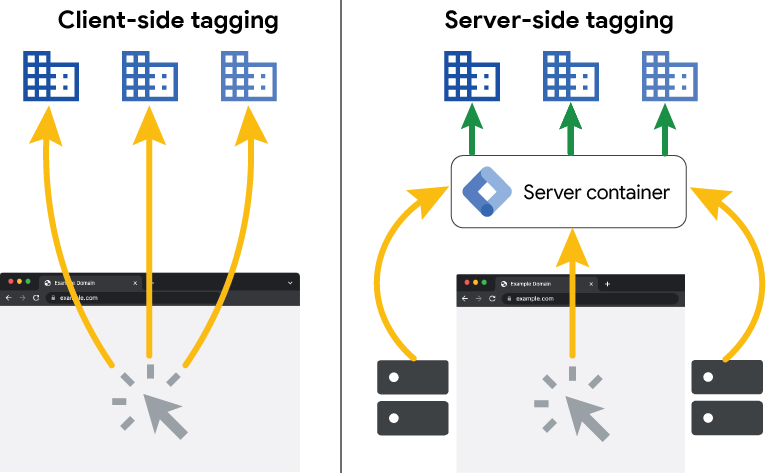
Example:
An ecommerce company transitions to server-side tagging to retain high-quality data even when technologies like Safari’s Intelligent Tracking Prevention (ITP) break JavaScript-based tracking.
As a result, the advertiser sees a complete picture of all the conversions driven by digital marketing and can now justify higher bids, which makes them more competitive in the ad auction and boosts total sales for their brand.
Actionable Tips:
- Implement GA4 Consent Mode and server-side tagging to maintain accurate performance data.
- Leverage data clean rooms to analyze cross-platform conversions securely.
- Use modeled conversions to fill tracking gaps caused by privacy restrictions.
2. Extract Data Insights And Make Smarter Decisions
Now that we’ve covered technologies that can stem the decline in access to data, let’s examine how AI can help make sense of it all.
The Problem With Data Analysis Before AI
Marketers may struggle to extract actionable insights when looking at a mountain of PPC data.
Humans simply aren’t as good as machines at detecting patterns or spotting anomalies in large data sets.
While statistical methods have long been used to find these patterns, many marketing teams lack the expertise to do it themselves or have no access to a qualified analyst to help them.
As a result, teams miss opportunities or spend more time than they can afford looking for signals to guide optimization efforts.
AI Solutions For Data Analysis And Attribution
1. Data-Driven Attribution Models (DDA)
DDA isn’t the newest solution in attribution modeling, but it exists largely because AI has become cheaper and more accessible.
It solves the problem of assigning values to different parts of the consumer journey when users take a multitude of paths from discovery to purchase.
Static attribution models lack the sophistication to account for this and cause advertisers to bid incorrectly.
Google’s data-driven attribution (DDA) uses machine learning to analyze conversion paths and assign credit based on a more complete analysis of a user’s consumer journey.
Unlike static models, DDA dynamically adjusts credit allocation to reflect the many ways consumers behave.
Machine learning, a form of AI, is what enabled Google to make this more advanced attribution model available to all advertisers and what has driven the steady improvement in results from Smart Bidding.
2. Automating Auction Insights Visualization
Generative AI is not only enhancing attribution but also automating repetitive tasks.
Recently, I tested GPT Operator to streamline several PPC reporting workflows.
Operator is OpenAI’s tool that lets the AI use a web browser to achieve tasks. It goes beyond searching on the web; it allows you to follow links, fill in forms, and interact intelligently with websites.
In one task, I asked Operator to download auction insights, visualize the data using Optmyzr’s Auction Insights Visualizer, and email a report.
It handled the data transfer and visualization steps flawlessly, though it struggled with taking a clean screenshot instead of attempting to attach HTML.
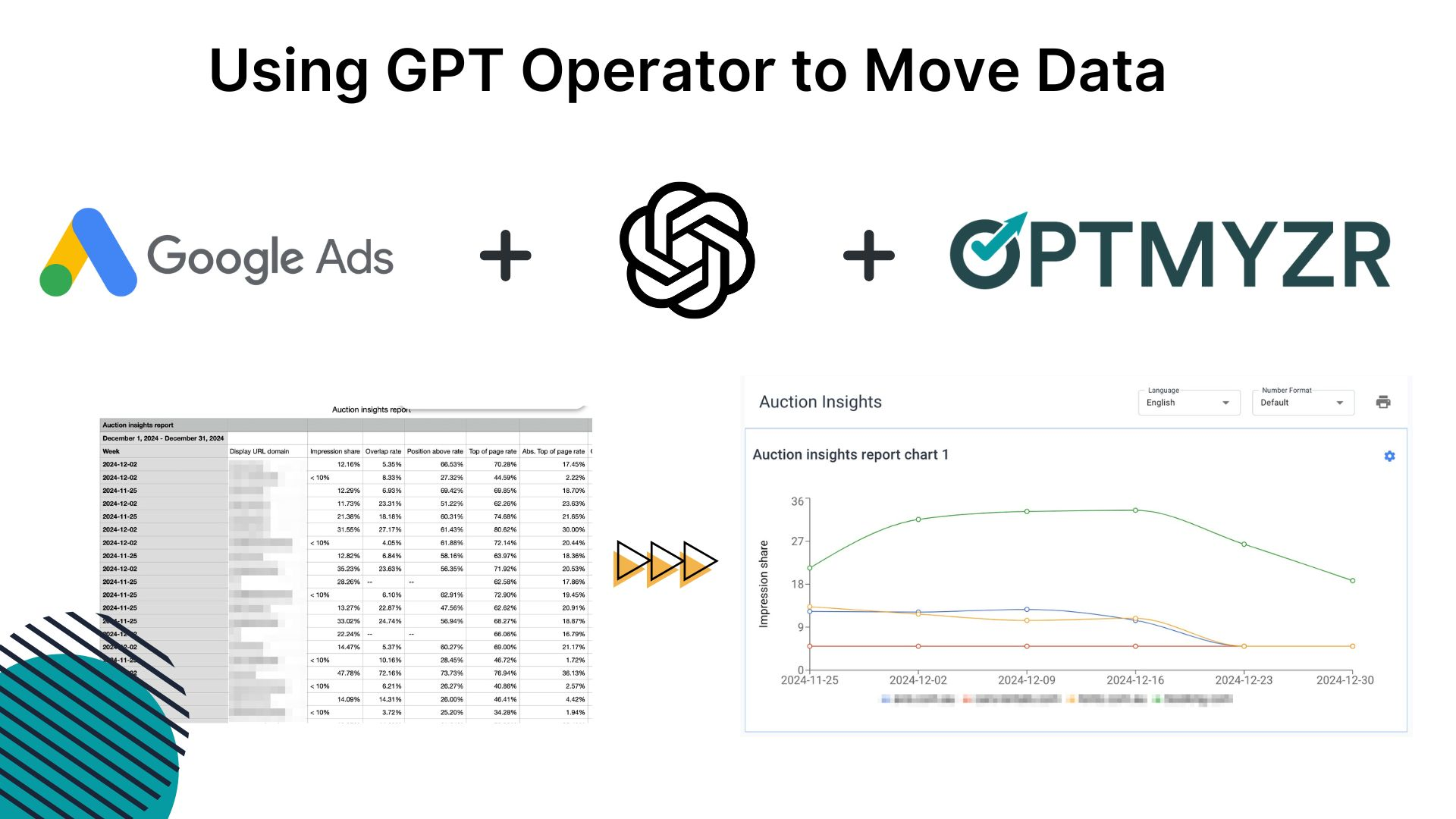
This illustrates how AI agents can help when data lives in disparate places. There are no APIs available to move it, as is the case with auction insights data from Google.
While Operator still needs too much hand-holding to be helpful today, it seems likely that we’re less than a year away from when it can do many tedious tasks for us.
3. Advanced Statistical Analysis Available To Anyone
Before AI advancements, conducting a statistical analysis could be a labor-intensive process requiring specialized software or data science expertise.
But today, generative AI enables marketers to explore these areas that were previously firmly outside their realm of expertise.
For example, GPT can explain and execute a process like a seasonality decomposition. AI can quickly write Python code that breaks down campaign data into trend, seasonal, and residual components, helping marketers uncover patterns they can act on.
How AI Automates Seasonal Analysis
In one of my PPC Town Hall podcast episodes, Cory Lindholm demonstrated how GPT can handle complex seasonality analysis in minutes.
Inspired by this, I used GPT’s Advanced Data Analysis feature to upload weekly Google Ads data and run a full decomposition.
GPT efficiently cleaned the data, identified issues like formatting errors, and generated a breakdown of trends, seasonal variations, and residual fluctuations.
In the analysis, GPT flagged recurring trends, allowing me to pinpoint peak demand periods and optimize bid strategies ahead of time. Tasks that previously took hours now take just a few minutes.
On a side note, I have found large language models (LLMs) so helpful with coding that I am now using v0.dev almost weekly to create apps, browser extensions, and scripts on a weekly basis.
3. Communicate Results Effectively Across Teams
With solid data in place and AI-fueled ways to speed up analysis, we should have some great results to share with stakeholders.
But sharing results through reports has traditionally been one of the most time-consuming and least loved tasks that fall on the plate of the typical account manager. And there were other problems, too.
The Problem With Sharing Reports Before AI
Reports were often static, one-size-fits-all documents that failed to meet the needs of different stakeholders.
Executives required high-level summaries focused on ROI, marketing strategists needed cross-channel insights, and PPC specialists required detailed campaign data.
Customizing reports for each audience was time-consuming and prone to error.
AI Solutions For Tailored Reporting
1. LLM Report Summarization
LLMs such as Claude, Gemini, and ChatGPT can quickly generate different explanations of reports from the same underlying data, enabling efficient customization for each audience.
For example, ChatGPT can produce a concise executive summary alongside a more detailed keyword-level report for PPC teams.
But that customization can and should be taken even further. In OpenAI, it’s possible to create custom GPTs, each with its own instructions. This can be used to create a different ChatGPT flavor for every client.
Whereas today, agencies depend on their people to remember how each client likes to get their reports, GPT can be trained to remember these preferences.
Coisas como o quanto eles conhecem o PPC, que jargões eles costumam usar na empresa e até mesmo quais são as iniciativas estratégicas do ano.
Então, o LLM pode redigir o resumo de uma forma que ressoe com o leitor e até mesmo explicar como os resultados da campanha de marketing de busca são essenciais para os objetivos estratégicos da empresa para o ano.
2. Painéis interativos para transparência em tempo real
Painéis orientados por IA fornecem visualizações ao vivo e personalizáveis do desempenho da campanha. As partes interessadas podem explorar dados interativamente, filtrando por intervalos de datas, plataformas ou indicadores-chave de desempenho (KPIs), reduzindo a necessidade de atualizações manuais frequentes de relatórios.
E embora os painéis já existam há muito tempo, a IA pode ser usada para destacar rapidamente os insights mais relevantes.
Por exemplo, a AMC permite que os profissionais de marketing usem IA para gerar SQL para explorar os dados usando linguagem natural.
Na minha empresa, a Optmyzr, implantamos o Sidekick, que pode responder instantaneamente a perguntas sobre dados em qualquer conta, por exemplo, as maiores oportunidades de otimização ou vitórias no último mês.
Antes da IA, esses insights poderiam ter permanecido ocultos nos dados.
Dicas práticas:
- Configure GPTs personalizados para cada cliente com quem você trabalha.
- Implemente ferramentas de relatórios que usem linguagem natural para explorar os dados.
Conclusão: Da geração de relatórios à tomada de decisões estratégicas com IA generativa
A IA generativa redefiniu os relatórios de PPC, transformando um processo antes fragmentado e demorado em um fluxo de trabalho simplificado e baseado em insights.
Ele não apenas automatiza a coleta de dados e a geração de relatórios; ele também revela tendências ocultas, correlações e anomalias que, de outra forma, poderiam passar despercebidas.
Isso permite que os profissionais de marketing tomem decisões mais inteligentes, rápidas e estratégicas com base em insights em tempo real.
Com ferramentas baseadas em IA, os profissionais de marketing podem enxergar além das métricas superficiais, descobrindo padrões e oportunidades que os relatórios tradicionais levariam horas ou dias para revelar.
Essa melhor compreensão do desempenho capacita as equipes a refinar a alocação de orçamento, a estratégia criativa e a segmentação de campanha de forma mais eficaz, levando a resultados mais substanciais e maior lucratividade.
A conclusão é simples. Com a Generative AI, os gerentes de PPC têm dados mais completos, levando a melhores insights e melhores decisões – tudo isso pode ser compartilhado de forma mais significativa com todos os stakeholders envolvidos.
Mais recursos:
Imagem em destaque: Igor Link/Shutterstock
#Maneiras #de #Mudar #PPC #Relatórios